With the potential annual value of AI and analytics for global banking estimated to reach $1 trillion,1 financial institutions are seeking out efficient ways to implement insights-driven lending. As regulators continue to supervise risk management, lenders must balance the opportunity presented by AI to determine risk more accurately while growing approval rates and reducing the cost of acquisition, with the ability to explain decisions.
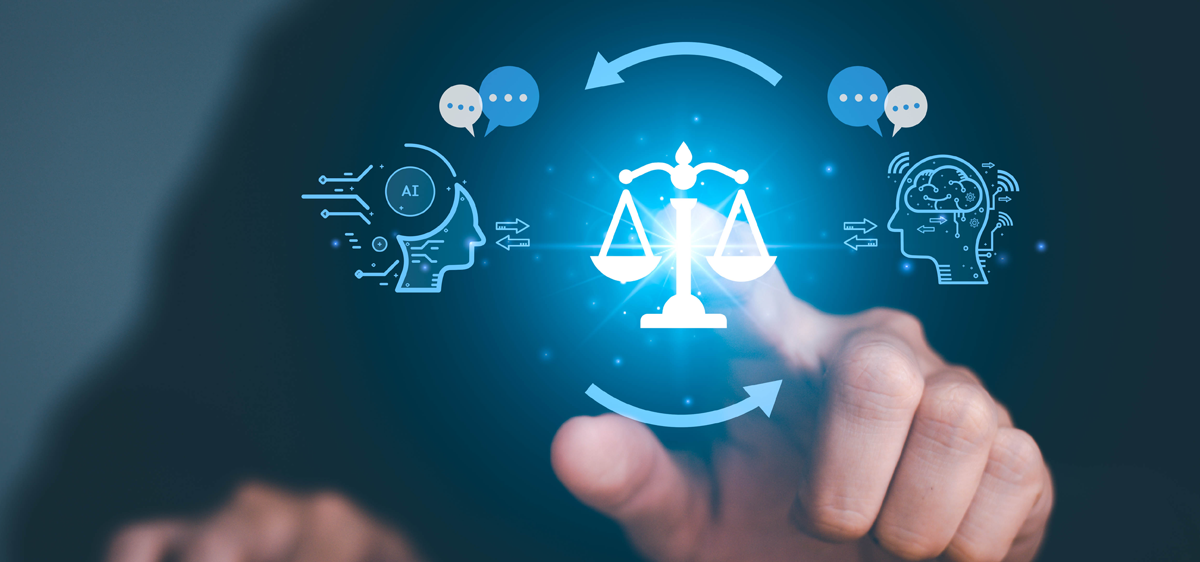
The challenge of using AI in building credit risk models
In a recent study conducted by Forrester Consulting on behalf of Experian, the top pain points for technology decision makers in financial services were reported to be automation and availability of data.2 The implementation of accessible AI solutions in credit risk management allows businesses to improve efficiency and time-to-market metrics by widening data sources, improving automation and decreasing risk. But the implementation of AI and machine learning in credit risk models can pose other challenges.
The study also found that 31% of respondents felt that their organization could not clearly explain the reasoning behind credit decisions to customers.2 Although AI has been proven to improve the accuracy of predictive credit risk models, these advancements mean that many organizations need support in understanding and explaining the outcomes of AI-powered decisions to fulfil regulatory obligations, such as the Equal Credit Opportunity Act (ECOA).
Moving from traditional model development methodologies to Machine Learning (ML)
As lenders move away from traditional parametric models like logistic regression, to ML models like neural nets or tree-based ensemble methods, explainability becomes more complex. Logistic regression has for many years allowed for a clear understanding of the linear relationships between model attributes and the outcome (approval or decline). Once the model is estimated, it is completely explainable.
However, ML models are non-parametric, so there are no underlying assumptions made around the distribution (shape) of the sample. Furthermore, the relationships between attributes and outcomes are not assumed to be linear – they’re often non-linear and complex, involving interactions. Such models are perceived to be black boxes where data is consumed as an input, processed and a decision is made without any visibility around the inner dynamics of the model.
At the same time, it is possible for ML models to perform better when accurately classifying good customers and those deemed delinquent. Ensuring transparency and explainability is crucial – lenders must be able to identify and explain the most dominant attributes that contribute towards a decision to lend or not. They must also provide ‘reason codes’ at the customer level so any declined applicants can fully understand the main cause and have a path to remediation.
The importance of developing transparent and explainable models
By prioritizing the development of transparent and interpretable models, financial institutions can also better foster equitable lending practices. However, fair credit decisioning goes beyond the regulatory and ethical obligations – it also makes business sense. Unfair lending leads to higher default rates if creditworthiness is not accurately assessed, therefore increasing bad debts. Removing demographics considered to be the ‘unscored’ or ‘underserved’ (those who are credit worthy but do not have a traditional data trail, but instead a digital footprint comprised of alternative data) can also limit portfolio opportunity for businesses.
For these reasons, it is critical to remove or minimize model bias. Bias is an upstream issue that starts at the data collection stage and model algorithm selections. Models developed using logistic regression or machine learning algorithms can be made fairer through carefully selecting attributes relevant to credit decisioning and avoiding sensitive attributes like race, gender, or ethnicity. Wherever sensitive metrics are used, they should be down-weighted to suppress their impact on lending decisions. Some other techniques to mitigate bias include:
- Thoroughly reviewing the data samples used in modelling.
- Fair Model Training – Train models using fairness-aware techniques. This may involve adjusting the training process to penalise any discrimination that creeps in.
According to Forrester, an essential component of a decisioning platform is one that can “harness the power of AI while enhancing and governing it with well-proven and trusted human business expertise. The best automated decisions come from a combination of both.”3
Developing explainable models goes some way towards reducing bias, but making the decisions explainable to regulatory bodies is a separate issue, and in the digital age of AI, can require deep domain expertise to fulfil.
While AI-powered decisioning can help businesses make smarter decisions, they also need the ability to confidently explain their lending practices to stay compliant. With the help of an expert partner, organizations can gain an understanding of what contributed most to a decision and receive detailed and transparent documentation for use with regulators. This ensures lenders can safely grow approval rates, be more inclusive, and better serve their customers.
“The solution isn’t simply finding better ways to convey how a system works; rather, it’s about creating tools and processes that can help even the deep expert understand the outcome and then explain it to others.”
McKinsey: why businesses need explainable ai and how to deliver it
Experian’s Ascend Intelligence ServicesTM Acquire is a custom credit risk model development service that can better quantify risk, score more applicants, increase automation, and drive more profitable decisions.
Confidently explain lending practices:
Detailed, rigorous, and transparent documentation that has been proven to meet the strictest regulatory standards.
Breaking Machine Learning (ML) out of the black box:
Understand what contributed most to a decision and generate adverse action codes directly from the model through our patent-pending ML explainability.
References:
- “The executive’s AI playbook,” McKinsey.com. (See “Banking,” under “Value & Assess.”)
- In a study conducted by Forrester Consulting on behalf of Experian, we surveyed 660 and interviewed 60 decision makers for technology purchases that support the credit lifecycle at their financial services organisation. The study included businesses across North America, UK and Ireland, and Brazil.
- 2023_05_Forrester_AI-Decisioning-Platforms-Wave.pdf
- https://www.mckinsey.com/capabilities/quantumblack/our-insights/why-businesses-need-explainable-ai-and-how-to-deliver-it
Contributors:
Masood Akhtar, Global Product Marketing Manager