Lenders prioritise automation above all, according to research.
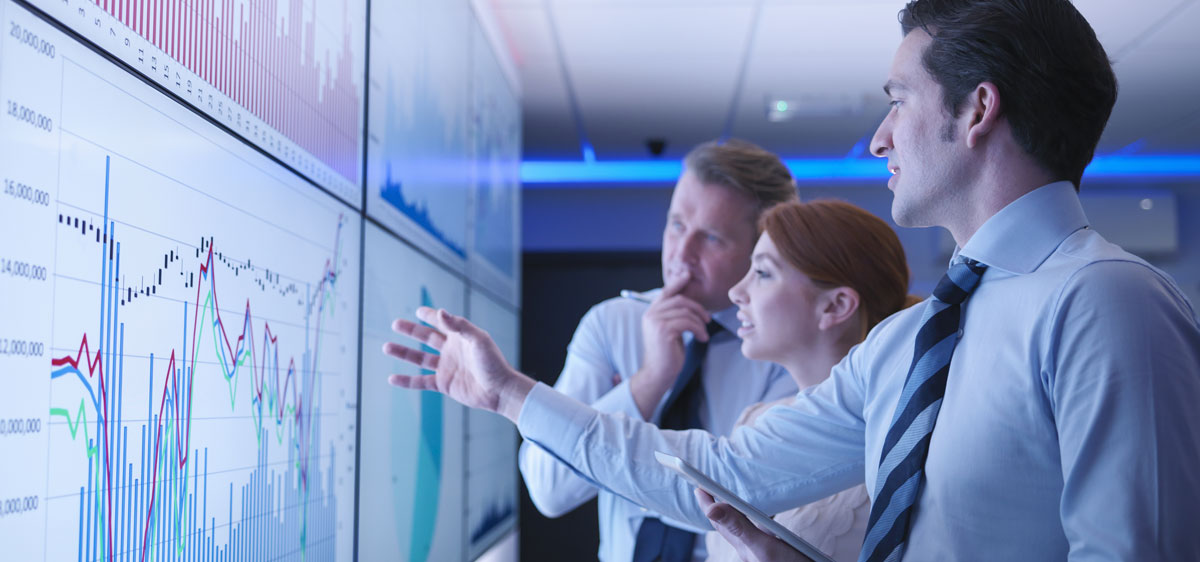
In a study conducted by Forrester Consulting on behalf of Experian, we surveyed 660 and interviewed 60 decision makers for technology purchases that support the credit lifecycle at their financial services organisation. The study included businesses across North America, UK and Ireland, and Brazil.
Research from Forrester on behalf of Experian found that automation is the top priority for businesses, and regardless of the specific industry or region, decision-makers consistently identified it as an important area of focus, and the biggest challenge. Lenders are using automation across the credit lifecycle and intend to invest further in the next 12 months, but there are multiple barriers to enhancing automation. We look at the use cases for automation and address the key challenges lenders face when automating decisions.
The automation agenda
The interpretation and application of automation vary hugely across the maturity spectrum of businesses in our research. While some companies consider automation as a means of simplifying tasks, such as the transition from manual processes to electronic spreadsheets, others are embracing its more advanced forms, such as AI-powered models.
Use cases for automation in lending
- Customer service chatbots using Natural Language Processing (NPL) combined with Robotic Process Automation system (RPA).
- Remote verification of customers using machine vision and RPA to cross-check data.
- Data governance – data cleansing of personal information from within data using RPA and NPL.
- Operational efficiencies using process mining and AI to identify automation opportunities.
- Credit and fraud risk decisioning, using machine learning.
Automation is about making processes as slick and robust as possible, giving the consumer a rapid journey so they can get processed very quickly, while behind the scenes lenders are making the best possible, compliant decisions, that protect them from losses around both credit risk and fraud.
Neil Stephenson, Vice President of Experian SOFTWARE SOLUTIONS CONSULTANCY
The changing face of automated decision-making in line with rapid tech advancements makes the use of automation by lenders a more complex opportunity than most. On one side there is the chance to enhance models with AI-powered tools to take away manual and subjective decision-making from processes. On the other, there’s the issue of governance and compliance – how to explain models that remove humans altogether.
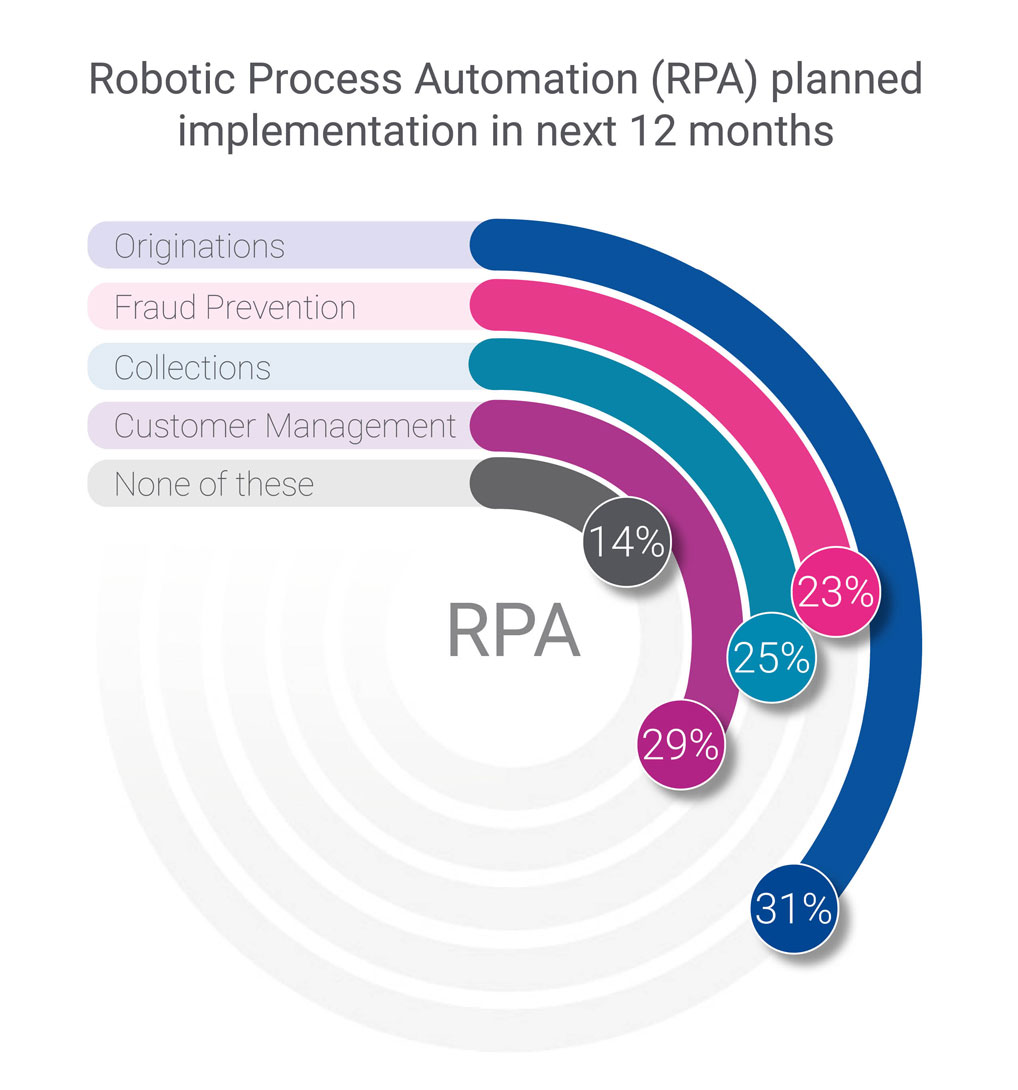
Introducing automation into some parts of the credit lifecycle isn’t always straightforward. Customer management has benefited from a lot of investment in the automation space over the years, particularly Natural Language Processing (NLP), but according to our research, the priority for business investment for Robotic Process Automation (RPA) in the next 12 months is originations. With onboarding playing such a key role in both customer experience and portfolio growth, businesses are looking to enhance this part of the credit lifecycle with automation.
Customer experience is driving growth
Automation plays a pivotal role in improving the customer journey and experience. The research showed that enhancing customer experience ranked even higher than growth as a priority for many organisations. As businesses strive to deliver seamless and personalised interactions, automation provides the necessary foundation for digital success, which in turn can strengthen competitiveness while retaining valuable customers.
“Strategically investing in automation offers businesses the opportunity to scale operations, with a primary focus on growth. In times of economic uncertainty, more targeted, customer-centric strategies, that encompass more accurate predictive models, built on up-to-date samples and executed rapidly, can help mitigate a higher-risk lending backdrop.” says Neil Stephenson, Vice President of Experian Software Solutions Consultancy. “Customer experience is the battleground for businesses, where they compete to deliver the best digital journeys in the market. It’s a battleground that isn’t just about increasing revenue – the market perception of an organisation can be as important as growth in some portfolios because businesses have a reputation to protect.”
Automating decisions can ensure customer experience is truly seamless, but businesses face multiple barriers when it comes to credit and risk decision automation.
Reducing referred applications
From scoring regression models to the development of machine learning models, better and smarter analytics are critical to drive the processes responsible for making application decisions. Reducing referred applications in turn decreases the need for manual intervention. By minimising the volume of applications in the middle of the credit score, lenders have a clearer and ultimately more automated approach to application accepts and declines. We interviewed decision-makers to understand the numerous challenges faced by lenders when automating decisions:
- Increasing data sources to allow for a more complete picture of the consumer
- Improved data quality, and increased volume of data
- Prevention of model bias
- The complexity of consumer type attached to some products
- Redundancy in data input and analytics
- Training across key roles for a better understanding of automation capabilities
- Explaining decisions based on machine learning models to regulators
- Complex fraud referral processes
For many respondents, automation is about accuracy and efficiency. By improving automation, there are fewer instances of errors and delays. To ensure scalability can exist in consistent, compliant, and accurate processes that work for both the business and the consumer, here are 10 tips to help tackle the challenges faced by lenders when it comes to automating decisions:
- Embrace advanced data aggregation tools and technologies that can efficiently collect and integrate data from various sources. Partner with known, trustworthy data providers to enrich datasets.
- Explore the use of no-code data management tools that allow users to add and remove data sources more quickly and easily.
- Implement data quality processes. Regularly audit and clean data to remove inconsistencies.
- Move to cloud-based solutions for scalable data storage and processing of very large datasets.
- Regularly audit (monitor) machine learning models for bias. Eliminating sampling bias is not yet possible but using a range of datasets (samples) and various sampling techniques will ensure representation across different demographics to help minimise bias.
- Develop specific models for different consumer segments or product categories. Regularly update models based on evolving consumer trends and behaviours.
- Conduct a thorough analysis of data inputs and streamline redundant variables. Use feature selection techniques such as correlations, weight of evidence, and information value to identify the most relevant information.
- Foster a culture of continuous learning and collaboration for all key stakeholders involved in the credit decisioning and strategy process.
- Develop transparent models with explainable features. Use interpretable machine learning algorithms that allow for clear explanations of decision factors at the customer level.
- Streamline identity verification processes by using smart orchestration to reduce false positives and prevent fraud.