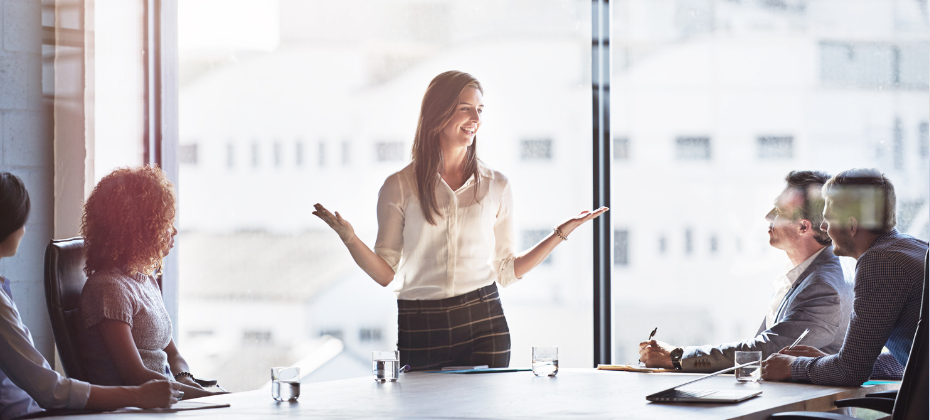
This article was updated on February 28, 2024.
There’s always a risk that a borrower will miss or completely stop making payments. And when lending is your business, quantifying that credit risk is imperative.
However, your credit risk analysts need the right tools and resources to perform at the highest level — which is why understanding the latest developments in credit risk analytics and finding the right partner are important.
What is credit risk analytics?
Credit risk analytics help turn historical and forecast data into actionable analytical insights, enabling financial institutions to assess risk and make lending and account management decisions. One way organizations do this is by incorporating credit risk modeling into their decisions.
Credit risk modeling
Financial institutions can use credit risk modeling tools in different ways.
They might use one credit risk model, also called a scorecard, to assess credit risk (the likelihood that you won’t be repaid) at the time of application. Its output helps you determine whether to approve or deny an application and set the terms of approved accounts.
Later in the customer lifecycle, a behavior scorecard might help you understand the risk in your portfolio, adjust credit lines and identify up- or cross-selling opportunities. Risk modeling can also go beyond individual account management to help drive high-level portfolio and strategic decisions.
However, managing risk models is an ongoing task. As market conditions and business goals change, monitoring, testing and recalibrating your models is important for accurately assessing credit risk.
Credit scoring models
Application credit scoring models are one of the most popular applications for credit risk modeling.
Designed to predict the probability of default (PD) when making lending decisions, conventional credit risk scoring models focus on the likelihood that a borrower will become 90 days past due (DPD) on a credit obligation in the following 24 months.
These risk scores are traditionally logistic regression models built on historical credit bureau data. They often have a 300 to 850 scoring range, and they rank-order consumers so people with higher scores are less likely to go 90 DPD than those with lower scores. However, credit risk models can have different score ranges and be developed to predict different outcomes over varying horizons, such as60 DPD in the next 12 months.
In addition to the conventional credit risk scores, organizations can use in-house and custom credit risk models that incorporate additional data points to better predict PD for their target market. However, they need to have the resources to manage the entire development and deployment or find an experienced partner who can help.
The latest trends in credit risk scoring
Organizations have used statistical and mathematical tools to measure risk and predict outcomes for decades. But the future of credit underwriting is playing out as big data meets advanced data analytics and increased computing power.
Some of the recent trends that we see are:
- Machine learning credit risk models: Machine learning (ML) is a type of artificial intelligence (AI) that’s proven to be especially helpful in evaluating credit risk. ML models can outperform traditional models by 10 to 15 percent.1 Experian survey data from September 2021 found that about 80 percent of businesses are confident in AI and cloud-based credit risk decisioning, and 70 percent frequently discuss using advanced analytics and AI for determining credit risk and collection efforts.2
- Expanding data sources: The ML models’ performance lift is due, in part, to their ability to incorporate internal and alternative credit data* (or expanded FCRA-regulated data), such as credit data from alternative financial services, rental payments and Buy Now Pay Later loans.
- Cognitively countering bias: Lenders have a regulatory and moral imperative to remove biases from their lending decisions. They need to beware of how biased training data could influence their credit risk models (ML or otherwise) and monitor the outcomes for unintentionally discriminatory results. This is also why lenders need to be certain that their ML-driven models are fully explainable — there are no black boxes.
- A focus on agility: The pandemic highlighted the need to have credit risk models and systems that you can quickly adjust to account for unexpected world events and changes in consumer behavior. Real-time analytical insights can increase accuracy during these transitory periods.
Financial institutions that can efficiently incorporate the latest developments in credit risk analytics have a lot to gain. For instance, a digital-first lending platform coupled with ML models allows lenders to increasingly automate loan underwriting, which can help them manage rising loan volumes, improve customer satisfaction and free up resources for other growth opportunities.
READ: The getting AI-driven decisioning right in financial services white paper to learn more about the current AI decisioning landscape.
Why does getting credit risk right matter?
Getting credit risk right is at the heart of what lenders do and accurately predicting the likelihood that a borrower won’t repay a loan is the starting point. From there, you can look for ways to more accurately score a wider population of consumers, and focus on how to automate and efficiently scale your system.
Credit risk analysis also goes beyond simply using the output from a scoring model. Organizations must make lending decisions within the constraints of their internal resources, goals and policies, as well as the external regulatory requirements and market conditions. Analytics and modeling are essential tools, but as credit analysts will tell you, there’s also an art to the practice.
CASE STUDY: Atlas Credit, a small-dollar lender, worked with Experian’s analytics experts to create a custom explainable ML-powered model using various data sources. After reworking the prequalification and credit decisioning processes and optimizing their score cutoffs and business rules, the company can now make instant decisions. It also doubled its approval rate while reducing risk by 15 to 20 percent.
How Experian helps clients
With decades of experience in credit risk analytics and data management, Experian offers a variety of products and services for financial services firms.
Ascend Intelligence Services™ is an award-winning, end-to-end suite of analytics solutions. At a high level, the offering set can rapidly develop new credit risk models, seamlessly deploy them into production and optimize decisioning strategies. It also has the capability to continuously monitor and retrain models to improve performance over time.
For organizations that have the experience and resources to develop new credit risk models on their own, Experian can give you access to data and expertise to help guide and improve the process. But there are also off-the-shelf options for organizations that want to quickly benefit from the latest developments in credit risk modeling.
1Experian (2020). Machine Learning Decisions in Milliseconds
2Experian (2021). Global Insights Report September/October 2021