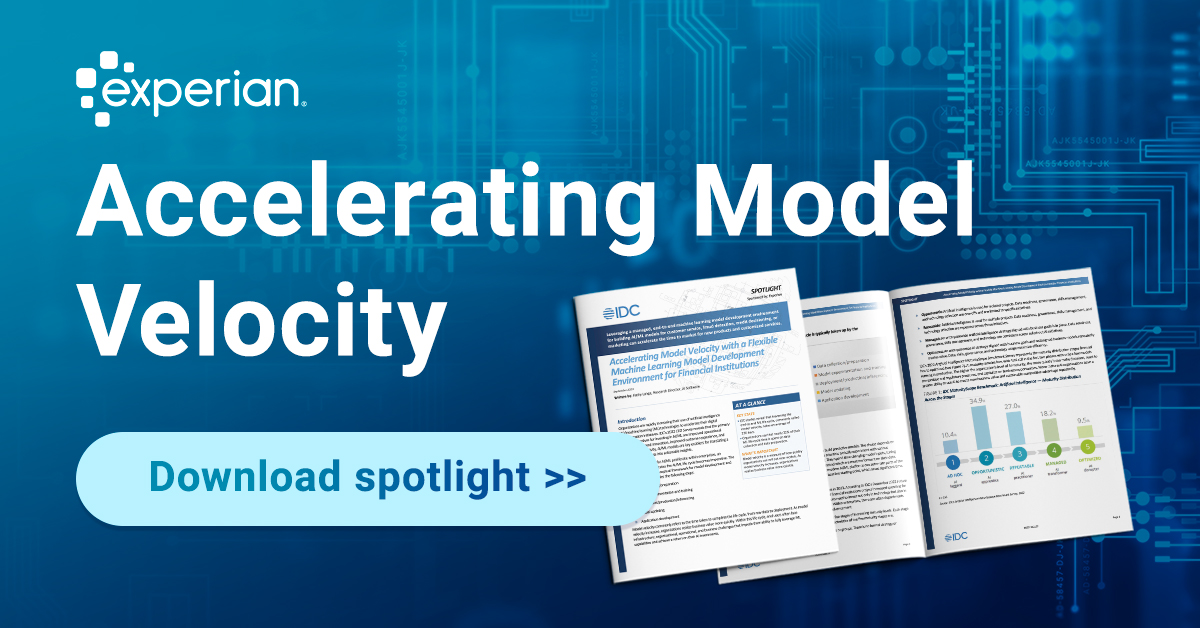
Data-driven machine learning model development is a critical strategy for financial institutions to stay ahead of their competition, and according to IDC, remains a strategic priority for technology buyers. Improved operational efficiency, increased innovation, enhanced customer experiences and employee productivity are among the primary business objectives for organizations that choose to invest in artificial intelligence (AI) and machine learning (ML), according to IDC’s 2022 CEO survey.
While models have been around for some time, the volume of models and scale at which they are utilized has proliferated in recent years. Models are also now appearing in more regulated aspects of the business, which demand increased scrutiny and transparency.
Implementing an effective model development process is key to achieving business goals and complying with regulatory requirements. While ModelOps, the governance and life cycle management of a wide range of operationalized AI models, is becoming more popular, most organizations are still at relatively low levels of maturity. It’s important for key stakeholders to implement best practices and accelerate the model development and deployment lifecycle.
Challenges impeding machine learning model development
Model development involves many processes, from wrangling data, analysis, to building a model that is ready for deployment, that all need to be executed in a timely manner to ensure proper outcomes. However, it is challenging to manage all these processes in today’s complex environment.
Modeling challenges include:
- Infrastructure: Necessary factors like storage and compute resources incur significant costs, which can keep organizations from evolving their machine learning capabilities.
- Organizational: Implementing machine learning applications requires talent, like data scientists and data and machine learning engineers.
- Operational: Piece meal approaches to ML tools and technologies can be cumbersome, especially on top of data being housed in different places across an organization, which can make pulling everything together challenging.
Opportunities for improvement are many
While there are many places where individuals can focus on improving model development and deployment, there are a few key places where we see individuals experiencing some of the most time-consuming hang-ups.
- Data wrangling and preparation
- Respondents to IDC’s 2022 AI StrategiesView Survey indicated that they spend nearly 22% of their time collecting and preparing data. Pinpointing the right data for the right purpose can be a big challenge. It is important for organizations to understand the entire data universe and effectively link external data sources with their own primary first party data. This way, stakeholders can have enough data that they trust to effectively train and build models.
- Model building
- While many tools have been developed in recent years to accelerate the actual building of models, the volume of models that often need to be built can be difficult given the many conflicting priorities for data teams within given institutions. Where possible, it is important for organizations to use templates or sophisticated platforms to ease the time to build a model and be able to repurpose elements that may already be working for other models within the business.
Improving Model Velocity
Experian’s Ascend ML BuilderTM is an on-demand advanced model development environment optimized to support a specific project. Features include a dedicated environment, innovative compute optimization, pre-built code called ‘Accelerators’ that simply, guide, and speed data wrangling, common analyses and advanced modeling methods with the ability to add integrated deployment.
To learn more about Experian’s Ascend ML Builder, click here.
To read the full Technology Spotlight, download “Accelerating Model Velocity with a Flexible Machine Learning Model Development Environment for Financial Institutions” here.
*This article includes content created by an AI language model and is intended to provide general information.