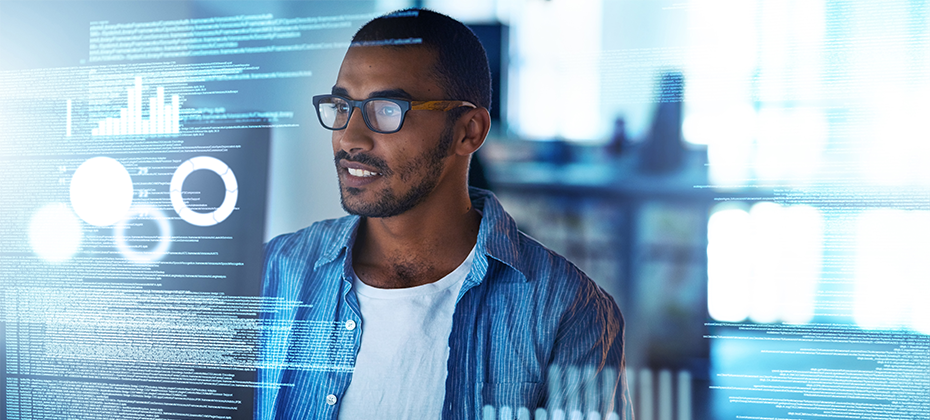
For businesses across all sectors solutions that improve productivity are more important than ever. As technology advances, organizations across industries are looking to capitalize by investing in artificial intelligence (AI) solutions. Studies have recently shown that productivity is a leading measure of how well these AI tools are performing. About 60% of organizations surveyed are using “improved productivity” as a metric to measure the success of implementing AI solutions.[1]
Experian research shows it takes an average of 15 months to build a model and put it into production. This can hinder productivity and the ability to quickly go to market. Without a deep understanding of key data points, organizations may also have difficulty realizing time to value efficiently.
To improve upon the modeling lifecycle, businesses must examine the challenges involved in the process.
The challenges of model building
One of the most significant challenges of the modeling lifecycle is speed. Slow modeling processes can cause delays and missed opportunities for businesses which they may have otherwise capitalized on. Another difficulty organizations face is having limited access to high-quality data to build more efficient models. Without the right data, businesses can miss out on actionable insights that could give them a competitive edge.
In addition, when organizations have inefficient resources, expenses can skyrocket due to the need for experts to intervene and address ongoing issues. This can result in a steep learning curve as new tools and platforms are adopted, making it difficult for organizations to operate efficiently without outside help.
Businesses can combat these challenges by implementing tools such as artificial intelligence (AI) to drive efficiency and productivity.
The AI journey
While generative AI and large language models are becoming more prevalent in everyday life, the path to incorporating a fully functional AI tool into an organization’s business operations involves multiple steps.
Beginning with a proof of concept, many organizations start their AI journey with building ideas and use cases, experimentation, and identifying and mitigating potential pitfalls, such as inaccurate or irrelevant information.
Once a proof of concept reaches an acceptable state of validity, organizations can move on to production and value at scale. During this phase, organizations will select specific use cases to move into production and measure their performance. Analyzing the results can help businesses glean valuable information about which techniques work most effectively, so they can apply those techniques to new use cases.
Following successful iterations of an efficiently functioning AI, the organization can then implement that AI as a part of their business by working the technology into everyday operations. This can help organizations drive productivity at scale across various business processes.
Experian’s AI journey has been ongoing, with years of expertise in implementing AI into various products and services. With a goal of providing greater insights to both businesses and consumers while adhering to proper consumer data privacy and compliance, Experian is committed to responsibly using AI to combat fraud and foster greater financial access and inclusion.
Our most recent AI innovation, Experian Assistant, is redefining how financial organizations improve productivity with data-driven insights.
Introducing Experian Assistant
Experian Assistant, a new GenAI tool announced in October at Money20/20 in Las Vegas, is helping organizations take their productivity to the next level by drastically speeding up the modeling lifecycle.
To drive automation and greater intelligence for Experian partners, Experian Assistant enables users to interact with a virtual assistant in real time and offers customized guidance and code generation for our suite of software solutions.
Our experts – Senior Director of Product Management Ankit Sinha and Director of Analyst Relations Erin Haselkorn – recently revealed the details of how Experian Assistant can cut down model-development timelines from months to days, and in some cases even hours. The webinar, which took place on November 7th, covered a wide range of features and benefits of the new tool, including:
- Spending less time writing code
- Enhancing understanding of data and attributes
- Accelerating time to value
- Improving regulatory compliance
A case study in building models faster
Continental Finance Company, LLC’s Chief Data Scientist shared their experience using Experian Assistant and how it has improved their organization’s modeling capabilities:
“With Experian Assistant, there is a lot of efficiency and improvement in productivity. We have reduced the time spent on data building by almost 75%, so we can build a model much quicker, and the code being generated by Experian Assistant is very high quality, enabling us to move forward much faster.”
For businesses looking to accelerate their modeling lifecycle and move more quickly with less effort, Experian Assistant provides a unique opportunity to significantly improve productivity and efficiency.
Experian Assistant tech showcase
Did you miss the Experian Assistant Tech Showcase webinar? Watch it on demand here and visit our website to learn more.
[1] Forrester’s Q2 AI Pulse Survey, 2024