In this article…
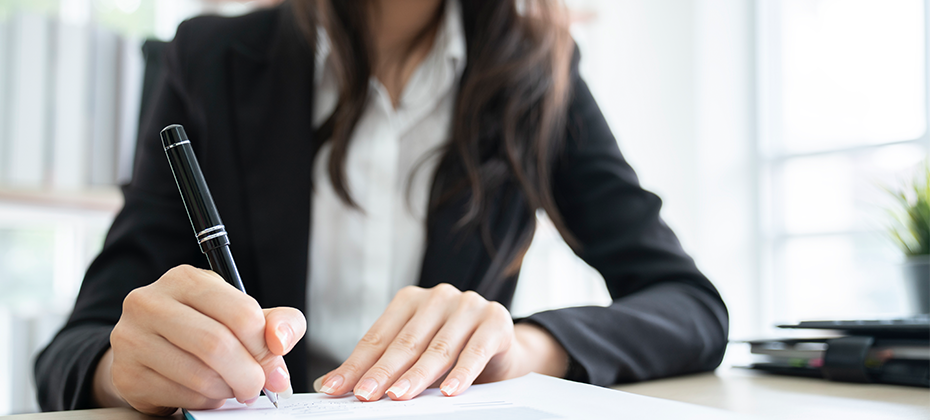
Dealing with delinquent debt is a challenging yet crucial task, and when faced with economic uncertainties, the need for effective debt management and collection strategies becomes even more pressing. Thankfully, advanced analytics offers a promising solution. By leveraging data-driven insights, you can enhance operational efficiency, better prioritize accounts, and make more informed decisions. This article explores how advanced analytics can revolutionize debt collection and provides actionable strategies to implement treatment.
Understanding advanced analytics in debt collection
Advanced analytics involves using sophisticated techniques and tools to analyze complex datasets and extract valuable insights. In debt collection, advanced analytics can encompass various methodologies, including predictive modeling, machine learning (ML), data mining, and statistical analysis.
Predictive modeling
Predictive modeling leverages historical data to forecast future outcomes. By applying predictive models to debt collection, you can estimate each account’s repayment likelihood. This helps prioritize your efforts toward accounts with a higher chance of recovery.
Machine learning
Machine learning algorithms can automatically identify patterns in large datasets, enabling more accurate predictions and classifications. For debt collectors, this means better segmenting delinquent accounts based on likelihood of repayment, risk, and customer behavior.
Data mining
Data mining involves exploring large datasets to unearth hidden patterns and correlations. In debt collection, data mining can reveal previously unnoticed trends and behaviors, allowing you to tailor your strategies accordingly.
Statistical analysis
Statistical methods help quantify relationships within data, providing a clearer picture of the factors influencing debt repayment and focusing on statistically significant repayment drivers, which aids in refining collection strategies.
Benefits of advanced analytics in delinquent debt collection
The benefits of employing advanced analytics in delinquent debt collection are multifaceted and valuable. By integrating these technologies, financial institutions can achieve greater efficiency, reduce operational costs, and improve recovery rates.
Enhanced prioritization and decisioning
With data and predictive analytics, you can gain a complete view of existing and potential customers to determine risk exposure and prioritize accounts effectively. By analyzing payment histories, credit scores, and other consumer behavior, you can enhance your collections prioritization strategies and focus on accounts more likely to pay or settle. This ensures that resources are allocated efficiently, and decisions are informed, maximizing your return on investment.
Tech showcase
In our recent tech showcase, experts explore how to harness the power of our industry-leading collection decisioning and optimization capabilities.
Watch Now
Reduced costs
Advanced analytics can significantly reduce operational costs by streamlining the collection process and targeting accounts with higher recovery potential. Automated processes and optimized resource allocation mean you can achieve more with less, ultimately increasing profitability.
Better customer relationships
With debt collection analytics, digital communication tools, artificial intelligence (AI), and ML processes, you can enhance your collections efforts, allowing you to better engage with consumers and increase response rates. Adopting a more empathetic and customer-centric approach that embraces omnichannel collections can foster positive customer relationships.
Implementing advanced analytics: A step-by-step guide
Step 1: Data collection and integration
The first step in implementing advanced analytics is to gather and integrate data from various sources. This includes payment histories, account information, demographic data, and external data such as credit scores. Ensuring data quality and consistency is crucial for accurate analysis.
Step 2: Data analysis and modeling
Once the data is collected, the next step is to apply advanced analytical techniques. This involves developing predictive models, training machine learning algorithms, and conducting statistical analyses to identify notable patterns and trends.
Step 3: Strategy development
Based on the insights gained from the analysis, you can develop targeted collection strategies. These may include segmenting accounts, prioritizing high-potential recoveries, and choosing the most effective communication methods. It’s essential to test and refine these strategies to ensure optimal performance continually.
Step 4: Automation and implementation
Implementing advanced analytics often involves automation. Workflow automation tools can streamline routine tasks, ensuring strategies are executed consistently and efficiently. Integrating these tools with existing debt collection systems can enhance overall effectiveness.
Step 5: Monitoring and optimization
Finally, continuously monitor the performance of your advanced analytics initiatives. Use key performance indicators (KPIs) to track success and identify areas for improvement. Regularly update models and strategies based on new data and evolving trends to maintain high recovery rates.
Putting it all together
Advanced analytics hold immense potential for transforming delinquent debt collection and can drive better return on investment. By leveraging predictive modeling, machine learning, data mining, and statistical analysis, financial institutions and debt collection agencies can perfect their collection best practices, prioritize accounts effectively, and make more informed decisions.
Our debt collection analytics and recovery tools empower your organization to see the complete behavioral, demographic, and emerging view of customer portfolios through extensive data assets, advanced analytics, and platforms. As the financial landscape evolves, working with an expert to adopt advanced analytics will be critical for staying competitive and achieving sustainable success in debt collection.
*This article includes content created by an AI language model and is intended to provide general information.