In this article…
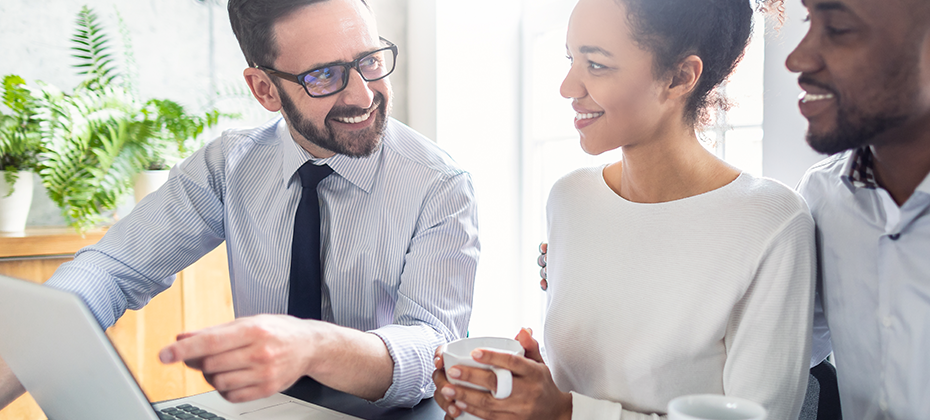
As the financial sector continues to embrace technological innovations, machine learning models are becoming indispensable tools for credit decisioning. These models offer enhanced efficiency and predictive power, but they also introduce new challenges. These challenges particularly concern fairness and bias, as complex machine learning models can be difficult to explain. Understanding how to ensure fair lending practices while leveraging machine learning models is crucial for organizations committed to ethical and compliant operations.
What is fair lending?
Fair lending is a cornerstone of ethical financial practices, prohibiting discrimination based on race, color, national origin, religion, sex, familial status, age, disability, or public assistance status during the lending process. This principle is enshrined in regulations such as the Equal Credit Opportunity Act (ECOA) and the Fair Housing Act (FHA). Overall, fair lending is essential for promoting economic opportunity, preventing discrimination, and fostering financial inclusion.
Key components of fair lending include:
- Equal treatment: Lenders must treat all applicants fairly and consistently throughout the lending process, regardless of their personal characteristics. This means evaluating applicants based on their creditworthiness and financial qualifications rather than discriminatory factors.
- Non-discrimination: Lenders are prohibited from discriminating against individuals or businesses on the basis of race, color, religion, national origin, sex, marital status, age, or other protected characteristics. Discriminatory practices include redlining (denying credit to applicants based on their location) and steering (channeling applicants into less favorable loan products based on discriminatory factors).
- Fair credit practices: Lenders must adhere to fair and transparent credit practices, such as providing clear information about loan terms and conditions, offering reasonable interest rates, and ensuring that borrowers have the ability to repay their loans.
- Compliance: Financial institutions are required to comply with fair lending laws and regulations, which are enforced by government agencies such as the Consumer Financial Protection Bureau (CFPB) in the United States. Compliance efforts include conducting fair lending risk assessments, monitoring lending practices for potential discrimination, and implementing policies and procedures to prevent unfair treatment.
- Model governance: Financial institutions should establish robust governance frameworks to oversee the development, implementation and monitoring of lending models and algorithms. This includes ensuring that models are fair, transparent, and free from biases that could lead to discriminatory outcomes.
- Data integrity and privacy: Lenders must ensure the accuracy, completeness, and integrity of the data used in lending decisions, including traditional credit and alternative credit data. They should also uphold borrowers’ privacy rights and adhere to data protection regulations when collecting, storing, and using personal information.
Understanding machine learning models and their application in lending
Machine learning in lending has revolutionized how financial institutions assess creditworthiness and manage risk. By analyzing vast amounts of data, machine learning models can identify patterns and trends that traditional methods might overlook, thereby enabling more accurate and efficient lending decisions. However, with these advancements come new challenges, particularly in the realms of model risk management and financial regulatory compliance. The complexity of machine learning models requires rigorous evaluation to ensure fair lending. Let’s explore why.
The pitfalls: bias and fairness in machine learning lending models
Despite their advantages, machine learning models can inadvertently introduce or perpetuate biases, especially when trained on historical data that reflects past prejudices. One of the primary concerns with machine learning models is their potential lack of transparency, often referred to as the “black box” problem.
Model explainability aims to address this by providing clear and understandable explanations of how models make decisions. This transparency is crucial for building trust with consumers and regulators and for ensuring that lending practices are fair and non-discriminatory.
Fairness metrics
Key metrics used to evaluate fairness in models can include standardized mean difference (SMD), information value (IV), and disparate impact (DI). Each of these metrics offers insights into potential biases but also has limitations.
- Standardized mean difference (SMD). SMD quantifies the difference between two groups’ score averages, divided by the pooled standard deviation. However, this metric may not fully capture the nuances of fairness when used in isolation.
- Information value (IV). IV compares distributions between control and protected groups across score bins. While useful, IV can sometimes mask deeper biases present in the data.
- Disparate impact (DI). DI, or the adverse impact ratio (AIR), measures the ratio of approval rates between protected and control classes. Although DI is widely used, it can oversimplify the complex interplay of factors influencing credit decisions.
Regulatory frameworks and compliance in fair lending
Ensuring compliance with fair lending regulations involves more than just implementing fairness metrics. It requires a comprehensive end-to-end approach, including regular audits, transparent reporting, and continuous monitoring and governance of machine learning models. Financial institutions must be vigilant in aligning their practices with regulatory standards to avoid legal repercussions and maintain ethical standards.
Read more: Journey of a machine learning model
How Experian® can help
By remaining committed to regulatory compliance and fair lending practices, organizations can balance technological advancements with ethical responsibility. Partnering with Experian gives organizations a unique advantage in the rapidly evolving landscape of AI and machine learning in lending. As an industry leader, Experian offers state-of-the-art analytics and machine learning solutions that are designed to drive efficiency and accuracy in lending decisions while ensuring compliance with regulatory standards.
Our expertise in model risk management and machine learning model governance empowers lenders to deploy robust and transparent models, mitigating potential biases and aligning with fair lending practices. When it comes to machine learning model explainability, Experian’s clear and proven methodology assesses the relative contribution and level of influence of each variable to the overall score — enabling organizations to demonstrate transparency and fair treatment to auditors, regulators, and customers.
Interested in learning more about ensuring fair lending practices in your machine learning models?
This article includes content created by an AI language model and is intended to provide general information.