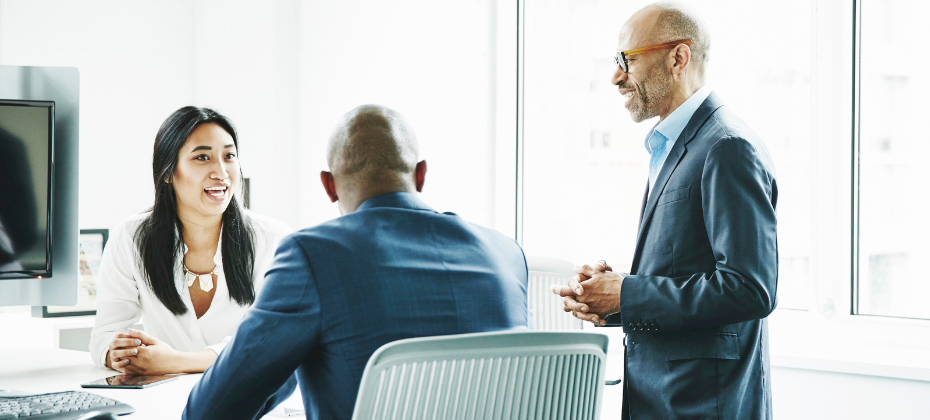
From chatbots to image generators, artificial intelligence (AI) has captured consumers’ attention and spurred joy — and sometimes a little fear. It’s not too different in the business world. There are amazing opportunities and lenders are increasingly turning to AI-driven lending decision engines and processes. But there are also open questions about how AI can work within existing regulatory requirements, how new regulations will impact its use and how to implement advanced analytics in a way that increases equitable inclusion rather than further embedding disparities.
How are lenders using AI today?
Many financial institutions have embraced — or at least tested — AI within several parts of their organization. The most advanced use of machine learning (ML) models tends to occur with credit card and unsecured personal loanunderwriting.1However, by late 2021, nearly three-quarters of businesses had used AI and machine learning, and 81 percent felt confident in using advanced analytics and AI incredit risk decisioning.2
READ MORE: AI and Machine Learning for Financial Institutions
Today, lenders are implementing AI-driven tools throughout the customer lifecycle to:
- Target the right consumers: Lenders can sift through vast amounts of data to find consumers who match their credit criteria and send right-sized offers, which enables them to maximize their acceptance rates.
- Detect and prevent fraud: Fraud detection tools have used AI and machine learning techniques to detect and prevent fraud for years. These systems may be even more important as fraudsters invest in technology and conduct increasingly sophisticated attacks.
- Assess creditworthiness: Machine learning-based models can incorporate a range of internal and external data points to more precisely evaluate creditworthiness and create a 10 to 15 percent performance lift compared with traditional linear and logistic regression models.3
- Automate decisions: More precise evaluations can increase how many applications flow into your automated approval and denial process rather than requiring a manual review.
- Manage portfolios: Lenders can also use a more complete picture of their current customers to make better decisions. For example, AI-driven models can help lenders set initial credit limits and suggest when a change could help them increase wallet share or reduce risk. Lenders can also use AI to help determine which up- and cross-selling offers to present and when (and how) to reach out.
- Improve collections: Models can be built to ease debt collection processes, such as choosing where to assign accounts, which accounts to prioritize and how to contact the consumer.
Additionally, businesses around the world have recognized improving customer acquisition and digital engagement as top priorities. In a recent Experian survey, companies ranked investing in AI second, behind investing in decisioning software, as the best way to improve their digital experiences.2
The benefits of AI in lending
Although lenders can use machine learning models in many ways, the primary drivers for adoption in underwriting are:1
- Improving credit risk assessment
- Faster development and deployment cycles for new or recalibrated models
- Unlocking the possibilities within large datasets
- Keeping up with competing lenders
Some of the use cases for machine learning solutions have a direct impact on the bottom line — improving credit risk assessment can decrease charge-offs. Others are less direct but still meaningful. For instance, machine learning models might increase efficiency and allow further automation. This takes the pressure off your underwriting team, even when application volume is extremely high, and results in faster decisions for applicants, which can improve your customer experience.
CASE STUDY: Atlas Credit, a small-dollar lender, used a machine learning-powered model and automation to nearly double its loan approval rates and decrease credit losses by up to 20 percent.
Incorporating large data sets into their decisions also allows lenders to expand their lending universe without taking on additional risk. For example, they may now be able to offer risk-appropriate credit lines to consumers that traditional scoring models can’t score.
And machine learning solutions can increasecustomer lifetime value when they’re incorporated throughout the customer lifecycle by stopping fraud, improving retention, increasing up- or cross-selling and streamlining collections.
Hurdles to adoption of machine learning in lending
There are clear benefits and interest in machine learning and analytics, but adoption can be difficult, especially within credit underwriting.
In August 2021, Forrester Consulting conducted a study commissioned by Experian and found the main barriers to adopting machine learning were:4
- Explainability of machine learning models (35 percent)
- Model deployment into decisioning strategy management systems (34 percent)
- Model deployment into live operational runtime environment (31 percent)
- Lack of access to in-house transactional data (30 percent)
- Lack of access to a wide range of traditional and non-traditional data assets (30 percent)
Explainability comes down to transparency and trust. Financial institutions have to trust that machine learning models will continue to outperform traditional models to make them a worthwhile investment. The models also have to be transparent and explainable for financial institutions to meet regulatory fair lending requirements.1
WATCH: Explainable Artificial Intelligence: The Case of Fair Lending
A lack of resources and expertise could hinder model development and deployment. It can take around nine months to build and deploy a custom model, and there’s a lot of overhead to cover during the process.5
Large lenders might have in-house credit modeling teams that can take on the workload, but they also face barriers when integrating new models into legacy systems. Small- and mid-sized institutions may be more nimble, but they rarely have the in-house expertise to build or deploy models on their own.
The models also have to be trained on appropriate data sets. Similar to model building and deployment, organizations might not have the human or financial resources to clean and organize internal data. And although vendors offer access to a lot of external data, sometimes sorting through and using the data requires a large commitment.
How Experian is shaping the future of AI in lending
Lenders are finding new ways to use AI throughout the customer lifecycle and with varying types of financial products. However, while the cost tocreate custom machine learning models is dropping,the complexities and unknowns are still too great for some lenders to manage. But that’s changing.5
Experian built the Ascend Intelligence Services™ to help smaller and mid-market lenders access the most advanced analytics tools. The managed service platform won a Fintech Breakthrough Award in 2021, and it can significantly reduce the cost and deployment time for lenders who want to incorporate AI-driven strategies and machine learning models into their lending process.
The end-to-end managed analytics service gives lenders access to Experian’s vast data sets and can incorporate internal data to build and seamlessly deploy custom machine learning models. The platform can also continually monitor and retrain models to increase lift, and there’s no “black box” to obscure how the model works. Everything is fully explainable, and theplatform bakesregulatory constraints into the data curation and model development to ensure lenders stay compliant.5
Learn more about our machine learning solutions.
* When we refer to “Alternative Credit Data,” this refers to the use of alternative data and its appropriate use in consumer credit lending decisions as regulated by the Fair Credit Reporting Act (FCRA). Hence, the term “Expanded FCRA Data” may also apply in this instance and both can be used interchangeably.
1FinRegLab (2021). The Use of Machine Learning for Credit Underwriting: Market & Data Science Context
2Experian (2021). Global Insights Report September/October 2021
3Experian (2020). Machine Learning Decisions in Milliseconds
4Experian (2022). Explainability: ML and AI in credit decisioning
5Experian (2021). Podcast: Advanced Analytics, Artificial Intelligence and Machine Learning in Lending