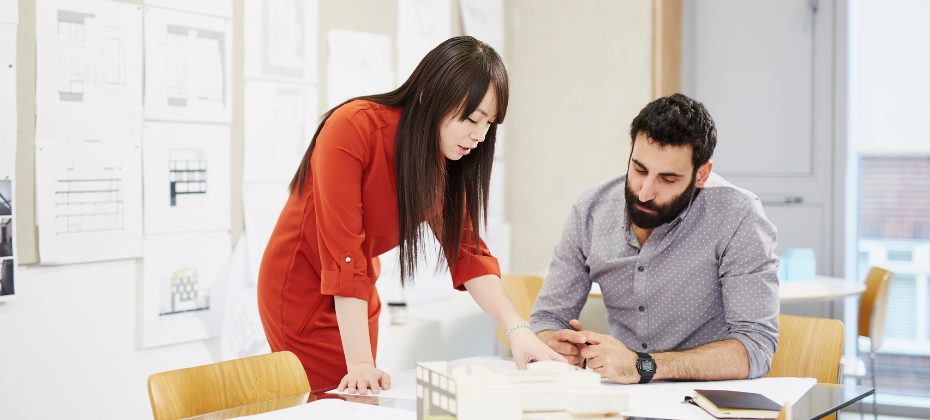
Developing machine learning (ML) credit risk models can be more challenging than traditional credit risk modeling approaches. But once deployed, ML models can increase automation and expand a lender’s credit universe.
For example, by using ML-driven credit risk models and combining traditional credit data with transactional bank data, a type of alternative credit data* , some lenders see a Gini uplift of 60 to 70 percent compared to a traditional credit risk model.1
New approaches to model operations are also helping lenders accelerate their machine learning model development processes and go from collecting data to deploying a new model in days instead of months.
READ MORE: Getting AI-driven decisioning right in financial services
What is machine learning model development?
Machine learning model development is what happens before the model gets deployed.It’s often broken down into several steps.
- Define the problem: If you’re building an ML credit risk model, the problem you may be trying to solve is anticipating defaults, improving affordability for borrowers or expanding your lending universeby scoring more thin-file and previously unscorable consumers.
- Gather, clean and stage data: Identify helpful data sources, such as internal, credit bureau and alternative credit data. The data will then need to be consolidated, structured, labeled and categorized. Machine learning can be useful here as well, as ML models can be trained to label and categorize raw data.
- Feature engineering: The data is then analyzed to identify the individual variables and clusters of variables that may offer the most lift. Features that may directly or unintentionally create bias should be removed or limited.
- Create the model: Deciding which algorithms and techniques to use when developing a model can be part art and part science. Because lenders need to be able to explain the decisions they make to consumers and regulators, many lenders build model explainability into new ML-driven credit risk models.
- Validate and deploy: New models are validated and rigorously tested, often as challengers to the existing champion model. If the new model can consistently outperform, it may move on to production.
The work doesn’t stop once a model is live — it needs to be continuously monitored for drift, and potentially recalibrated or replaced with a new model. About 10 percent of lenders use tools to automatically alert them when their models start to drift. But around half make a point of checking deployed models for drift every month or quarter.3
READ MORE: Journey of an ML Model
What is model deployment?
Model deployment is one of the final steps in the model lifecycle — it’s when you move the model from development and validation to live production.
New models can be deployed in various ways, including via API integration and cloud service deployment using public, private or hybrid architecture. However, integrating a new model with existing systems can be challenging.
About a third (33 percent) of consumer lending organizations surveyed in 2023 said it took them one to two months for model deployment-related activities. A little less (29 percent) said it took them three to six months.
Overall, it often takes up to 15 months for the entire development to deployment process — and 55 percent of lenders report building models that never get deployed.2
READ MORE: Accelerating the Model Development and Deployment Lifecycle
Benefits of deploying machine learning credit risk models
Developing, deploying, monitoring and recalibrating ML models can be difficult and costly. But financial institutions have a lot to gain from embracing the future of underwriting.
- Improve credit risk assessment: ML-driven models can incorporate more data sources and more precisely assess credit risk to help lenders price credit offers and decrease charge-offs.
- Expand automation: More precise scoring can also increase automation by reducing how many applications need to go to manual review.
- Increase financial inclusion: ML-models may be able to evaluate consumers who don’t have recent credit information or thick enough credit files to be scorable by traditional models.
In short, ML models can help lenders make better loan offers to more people while taking on less risk and using fewer internal resources to review applications.
CASE STUDY: Atlas Credit, a small-dollar lender, partnered with Experian® to develop a fully explainable machine learning credit risk model that incorporated internal data, trended data, alternative financial services data and Experian’s attributes. Atlas Credit can use the new model to make instant decisions and is expected to double its approvals while decreasing losses by up to 20 percent.
How we can help
Experian offers many machine learning solutions for different industries and use cases via the Experian Ascend Technology Platform™.
For example, with Ascend ML Builder™, lenders can access an on-demand development environment that can increase model velocity — the time it takes to complete a new model’s lifecycle. You can configure Ascend ML Builder based on the computeyou allocate and your use cases, and the included code templates (called Accelerators) can help with data wrangling, analysis and modeling.
There’s also Ascend Ops™, a cloud-based model operations solution. You can use Ascend Ops to register, test and deploy custom features and models. Automated model monitoring and management can also help you track feature and model data drift and model performance to improve models in production.
*When we refer to “Alternative Credit Data,” this refers to the use of alternative data and its appropriate use in consumer credit lending decisions, as regulated by the Fair Credit Reporting Act. Hence, the term “Expanded FCRA Data” may also apply and can be used interchangeably.
1. Experian (2023). Raising the AI Bar
2. Experian (2023). Accelerating Model Velocity in Financial Institutions
3. Ibid.