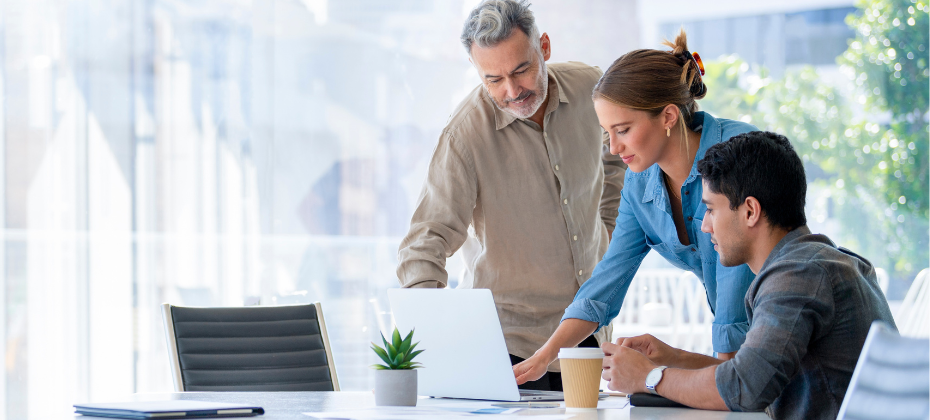
In this article…
In the lending world, making precise underwriting decisions is key to minimizing risks and optimizing returns. One valuable yet often overlooked technique that can significantly enhance your credit underwriting process is reject inferencing. This blog post offers insights into what reject inference is, how it can improve underwriting, and various reject inference methods.
What is reject inference?
Reject inference is a statistical method used to predict the potential performance of applicants who were rejected for a loan or credit — or approved but did not book. In essence, it helps lenders and financial institutions gauge how rejected or non-booked applicants might have performed had they been accepted or booked. By incorporating reject inference, you gain a more comprehensive view of the applicant pool, which leads to more informed underwriting decisions.
Utilizing reject inference helps reduce biases in your models, as decisions are based on a complete set of data, including those who were initially rejected. This technique is crucial for refining credit risk models, leading to more accurate predictions and improved financial outcomes.
How can reject inference enhance underwriting?
Incorporating reject inference into your underwriting process offers several advantages:
- Identifying high-potential customers: By understanding the potential behavior of rejected applicants, you can uncover high-potential customers who might have been overlooked before.
- Improved risk assessment: Considering the full spectrum of applicants provides a clearer picture of the overall risk landscape, allowing for more informed lending decisions. This can help reduce default rates and enhance portfolio performance.
- Optimizing credit decisioning models: Including inferred data from rejected and non-booked applicants makes your credit scoring models more representative of the entire applicant population. This results in more robust and reliable predictions.
Techniques in reject inference
Several techniques are employed in reject inference, each with unique strengths and applications. Understanding these techniques is crucial for effectively implementing reject inference in your underwriting process. Let’s discuss three commonly used techniques:
- Parceling: This technique involves segmenting rejected applicants based on their characteristics and behaviors, creating a more detailed view of the applicant pool for more precise predictions.
- Augmentation: This method adds inferred data to the dataset of approved applicants, producing a more comprehensive model that includes both approved and inferred rejected applicants, leading to better predictions.
- Reweighting: This technique adjusts the weights of approved applicants to reflect the characteristics of rejected applicants, minimizing bias towards the approved applicants and improving prediction accuracy.
Pre-diction method
The pre-diction method is a common approach in reject inference that uses data collected at the time of application to predict the performance of rejected applicants. The advantage of this method is its reliance on real-time data, making it highly relevant and current.
For example, pre-diction data can include credit bureau attributes from the time of application. This method helps develop a model that predicts the outcomes of rejected applicants based on performance data from approved applicants. However, it may not capture long-term trends and could be less effective for applicants with unique characteristics.
Post-diction method
The post-diction method uses data collected after the performance window to predict the performance of rejected applicants. Leveraging historical data, this method is ideal for capturing long-term trends and behaviors.
Post-diction data may include credit bureau attributes from the end of the performance window. This method helps develop a model based on historical performance data, which is beneficial for applicants with unique characteristics and can lead to higher performance metrics. However, it may be less timely and require more complex data processing compared to pre-diction.
Enhancing reject inference design for better classification
To optimize your reject inference design, focus on creating a model that accurately classifies the performance of rejected and non-booked applicants. Utilize a combination of pre-diction and post-diction data to capture both real-time and historical trends.
Start by developing a parceling model using pre-diction data, such as credit bureau attributes from the time of application, to predict rejected applicants’ outcomes. Regularly update your model with the latest data to maintain its relevance.
Next, incorporate post-diction data, including attributes from the end of the performance window, to capture long-term trends. Combining both data types will result in a more comprehensive model.
Consider leveraging advanced analytics techniques like machine learning and artificial intelligence to refine your model further, identifying hidden patterns and relationships for more accurate predictions.
How Experian can assist with reject inference
Reject inference is a powerful tool for enhancing your underwriting process. By predicting the potential performance of rejected and non-booked applicants, you can make more inclusive and accurate decisions, leading to improved risk assessment and optimized credit scoring models.
Experian offers various services and solutions to help financial institutions and lenders effectively implement reject inference into their decisioning strategy. Our solutions include comprehensive and high-quality datasets, which empower you to build models that are more representative of the entire applicant population. Additionally, our advanced analytics tools simplify data analysis and model development, enabling you to implement reject inference efficiently without extensive technical expertise.
Ready to elevate your underwriting process? Contact us today to learn more about our suite of advanced analytics solutions or hear what our experts have to say in this webinar.
This article includes content created by an AI language model and is intended to provide general information.