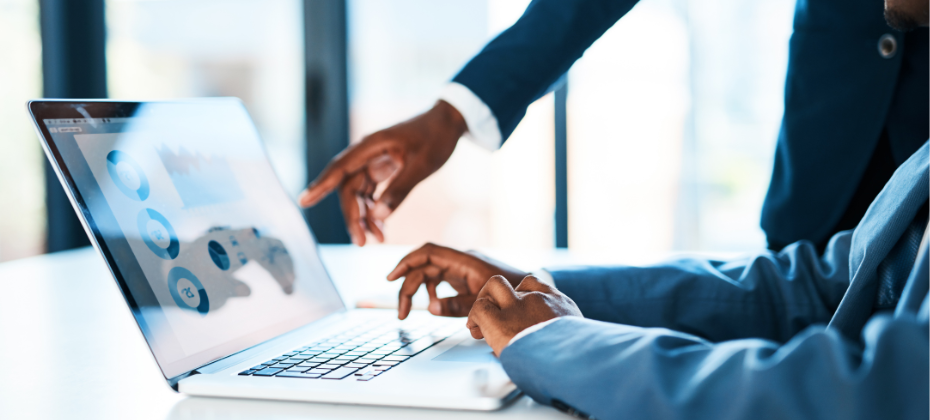
Model governance is growing increasingly important as more companies implement machine learning model deployment and AI analytics solutions into their decision-making processes. Models are used by institutions to influence business decisions and identify risks based on data analysis and forecasting. While models do increase business efficiency, they also bring their own set of unique risks. Robust model governance can help mitigate these concerns, while still maintaining efficiency and a competitive edge.
What is model governance?
Model governance refers to the framework your organization has in place for overseeing how you manage your development, model deployment, validation and usage.1 This can involve policies like who has access to your models, how they are tested, how new versions are rolled out or how they are monitored for accuracy and bias.2
Because models analyze data and hypotheses to make predictions, there’s inherent uncertainty in their forecasts.3 This uncertainty can sometimes make them vulnerable to errors, which makes robust governance so important. Machine learning model governance in banks, for example, might include internal controls, audits, a thorough inventory of models, proper documentation, oversight and ensuring transparent policies and procedures.
One significant part of model governance is ensuring your business complies with federal regulations. The Federal Reserve Board and the Office of the Comptroller of the Currency (OCC) have published guidance protocols for how models are developed, implemented and used. Financial institutions that utilize models must ensure their internal policies are consistent with these regulations. The OCC requirements for financial institutions include:
- Model validations at least once a year
- Critical review by an independent party
- Proper model documentation
- Risk assessment of models’ conceptual soundness, intended performance and comparisons to actual outcomes
- Vigorous validation procedures that mitigate risk
Why is model governance important — especially now?
More and more organizations are implementing AI, machine learning and analytics into their models. This means that in order to keep up with the competition’s efficiency and accuracy, your business may need complex models as well. But as these models become more sophisticated, so does the need for robust governance.3
Undetected model errors can lead to financial loss, reputation damage and a host of other serious issues. These errors can be introduced at any point from design to implementation or even after deployment via inappropriate usage of the model, drift or other issues. With model governance, your organization can understand the intricacies of all the variables that can affect your models’ results, controlling production closely with even greater efficiency and accuracy.
Some common issues that model governance monitors for include:2
- Testing for drift to ensure that accuracy is maintained over time.
- Ensuring models maintain accuracy if deployed in new locations or new demographics.
- Providing systems to continuously audit models for speed and accuracy.
- Identifying biases that may unintentionally creep into the model as it analyzes and learns from data.
- Ensuring transparency that meets federal regulations, rather than operating within a black box. Good model governance includes documentation that explains data sources and how decisions are reached.
Model governance use cases
Below are just three examples of use cases for model governance that can aid in advanced analytics solutions.
Credit scoring
A credit risk score can be used to help banks determine the risks of loans (and whether certain loans are approved at all). Governance can catch biases early, such as unintentionally only accepting lower credit scores from certain demographics. Audits can also catch biases for the bank that might result in a qualified applicant not getting a loan they should.
Interest rate risk
Governance can catch if a model is making interest rate errors, such as determining that a high-risk account is actually low-risk or vice versa. Sometimes changing market conditions, like a pandemic or recession, can unintentionally introduce errors into interest rate data analysis that governance will catch.
Security challenges
One department in a company might be utilizing a model specifically for their demographic to increase revenue, but if another department used the same model, they might be violating regulatory compliance.4Governance can monitor model security and usage, ensuring compliance is maintained.
Why Experian?
Experian® provides risk mitigation tools and objective and comprehensive model risk management expertise that can help your company implement custom models, achieve robust governance and comply with any relevant federal regulations. In addition, Experian can provide customized modeling services that provide unique analytical insights to ensure your models are tailored to your specific needs.
Experian’s model risk governance services utilize business consultants with tenured experience who can provide expert independent, third-party reviews of your model risk management practices. Key services include:
- Back-testing and benchmarking: Experian validates performance and accuracy, including utilizing statistical metrics that compare your model’s performance to previous years and industry benchmarks.
- Sensitivity analysis: While all models have some degree of uncertainty, Experian helps ensure your models still fall within the expected ranges of stability.
- Stress testing: Experian’s experts will perform a series of characteristic-level stress tests to determine sensitivity to small changes and extreme changes.
- Gap analysis and action plan: Experts will provide a comprehensive gap analysis report with best-practice recommendations, including identifying discrepancies with regulatory requirements.
Traditionally, model governance can be time-consuming and challenging, with numerous internal hurdles to overcome. Utilizing Experian’s business intelligence and analytics solutions, alongside its model risk management expertise, allows clients to seamlessly pass requirements and experience accelerated implementation and deployment.
Experian can optimize your model governance
Experian is committed to helping you optimize your model governance and risk management. Learn more here.
References
1Model Governance,” Open Risk Manual, accessed September 29, 2023. https://www.openriskmanual.org/wiki/Model_Governance
2Lorica, Ben, Doddi, Harish, and Talby, David. “What Are Model Governance and Model Operations?” O’Reilly, June 19, 2019. https://www.oreilly.com/radar/what-are-model-governance-and-model-operations/
3“Comptroller’s Handbook: Model Risk Management,” Office of the Comptroller of the Currency. August 2021. https://www.occ.treas.gov/publications-and-resources/publications/comptrollers-handbook/files/model-risk-management/pub-ch-model-risk.pdf
4Doddi, Harish. “What is AI Model Governance?” Forbes. August 2, 2021. https://www.forbes.com/sites/forbestechcouncil/2021/08/02/what-is-ai-model-governance/?sh=5f85335f15cd