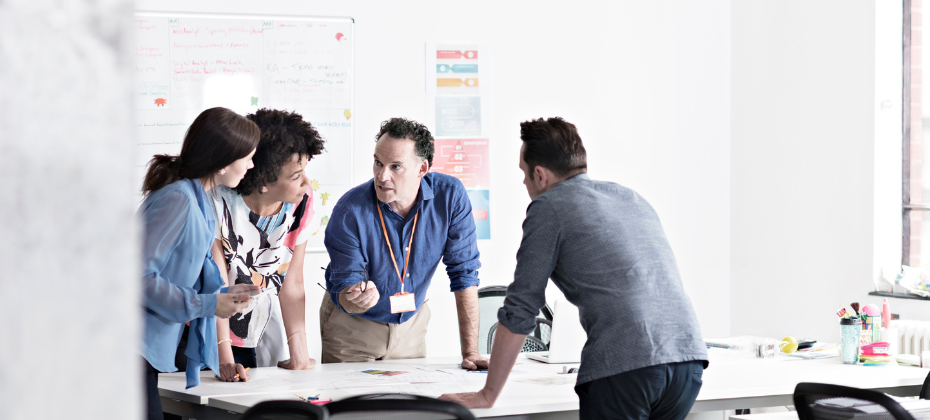
The science of turning historical data into actionable insights is far from magic. And while organizations have successfully used predictive analytics for years, we’re in the midst of a transformation.
New tools, vast amounts of data, enhanced computing power and decreasing implementation costs are making predictive analytics increasingly accessible. And business leaders from varying industries and functions can now use the outcomes to make strategic decisions and manage risk.
What is predictive analytics?
Predictive analytics is a type of data analytics that uses statistical modeling and machine learning techniques to make predictions based on historical data. Organizations can use predictive analytics to predict risks, needs and outcomes.
You might use predictive analytics to make an immediate decision. For example, whether or not to approve a new credit application based on a credit score — the output from a predictive credit risk model. But organizations can also use predictive analytics to make long-term decisions, such as how much inventory to order or staff to hire based on expected demand.
How can predictive business analytics help a business succeed?
Businesses can use predictive analytics in different parts of their organizations to answer common and critical questions. These include forecasting market trends, inventory and staffing needs, sales and risk.
With a wide range of potential applications, it’s no surprise that organizations across industries and functions are using predictive analytics to inform their decisions. Here are a few examples of how predictive analytics can be helpful:
- Financial services: Financial institutions can use predictive analytics to assess credit risk, detect fraudulent applicants or transactions, cross-sell customers and limit losses during recovery.
- Healthcare: Using data from health records and medical devices, predictive models can predict patient outcomes or identify patients who need critical care.
- Manufacturing: An organization can use models to predict when machines need to be turned off or repaired to improve their longevity and avoid accidents.
- Retail: Brick-and-mortar retailers might use predictive analytics when deciding where to expand, what to cross-sell loyalty program members and how to improve pricing.
- Hospitality: A large hospitality group might predict future reservations to help determine how much staff they need to hire or schedule.
Advanced techniques in predictive modeling for financial services
Emerging technologies, particularly AI and machine learning (ML), are revolutionizing predictive modeling in the financial sector by providing more accurate, faster and more nuanced insights. Taking a closer look at financial services, consider how an organization might use predictive credit analyticsand credit risk scores across the customer lifecycle.
- Marketing: Segment consumers to run targeted marketing campaigns and send prescreened credit offers to the people who are most likely to respond. AI models can analyze customer data to offer personalized offers and product recommendations.
- Underwriting: AI technologies enable real-time data analysis, which is critical for underwriting. The outputs from credit risk models can help you to quickly approve, deny or send applications for manual review. Explainable machine learning models may be able to expand automation and outperform predictive models built with older techniques by 10 to 15 percent.1 Fraud detection models can also raise red flags based on suspicious information or behaviors.
- Account management: Manage portfolios and improve customer retention, experience and lifetime value. The outputs can help you determine when you should adjust credit lines and interest rates or extend offers to existing customers. AI can automate complex decision-making processes by learning from historical data, reducing the need for human intervention and minimizing human error.
- Collections: Optimize and automate collections based on models’ predictions about consumers’ propensity to pay and expected recovery amounts. ML models, which are capable of processing vast amounts of unstructured data, can uncover complex patterns that traditional models might miss.
Although some businesses can use unsupervised or “black box” models, regulations may limit how financial institutions can use predictive analytics to make lending decisions. Fortunately, there are ways to use advanced analytics, including AI and ML, to improve performance with fully compliant and explainable credit risk models and scores.
WHITE PAPER: Getting AI-driven decisioning right in financial services
Developing predictive analytics models
Going from historical data to actionable analytics insights can be a long journey. And if you’re making major decisions based on a model’s predictions, you need to be confident that there aren’t any missteps along the way.
Internal and external data scientists can oversee the process of developing, testing and implementing predictive analytics models:
- Define your goal: Determine the predictions you want to make or problems you want to solve given the constraints you must act within.
- Collect data: Identify internal and external data sources that house information that could be potentially relevant to your goal.
- Prepare the data: Clean the data to prepare it for analysis by removing errors or outliers and determining if more data will be helpful.
- Develop and validate models: Create predictive models based on your data, desired outcomes and regulatory requirements. Deciding which tools and techniques to use during model development is part of the art that goes into the science of predictive analytics. You can then validate models to confirm that they accurately predict outcomes.
- Deploy the models: Once a model is validated, deploy it into a live environment to start making predictions. Depending on your IT environment, business leaders may be able to easily access the outputs using a dashboard, app or website.
- Monitor results: Test and monitor the model to ensure it’s continually meeting performance expectations. You may need to regularly retrain or redevelop models using training data that better reflects current conditions.
Depending on your goals and resources, you may want to start with off-the-shelf predictive models that can offer immediate insights. But if your resources and experience allow, custom models may offer more insights.
CASE STUDY: Experian worked with one of the largest retail credit card issuers to develop a custom acquisition model. The client’s goal was to quickly replace their outdated custom model while complying with their model governance requirements. By using proprietary attribute sets and a patented advanced model development process, Experian built a model that offered 10 percent performance improvements across segments.
Predictive modeling techniques
Data scientists can use different modeling techniques when building predictive models, including:
- Regression analysis: A traditional approach that identifies the most important relationships between two or more variables.
- Decision trees: Tree-like diagrams show potential choices and their outcomes.
- Gradient-boosted trees: Builds on the output from individual decision trees to train more predictive trees by identifying and correcting errors.
- Random forest: Uses multiple decision trees that are built in parallel on slightly different subsets of the training data. Each tree will give an output, and the forest can analyze all of these outputs to determine the most likely result.
- Neural networks: Designed to mimic how the brain works to find underlying relationships between data points through repeated tests and pattern recognition.
- Support vector machines: A type of machine learning algorithm that can classify data into different groups and make predictions based on shared characteristics.
Experienced data scientists may know which techniques will work well for specific business needs. However, developing and comparing several models using different techniques can help determine the best fit.
Implementation challenges and solutions in predictive analytics
Integrating predictive analytics into existing systems presents several challenges that range from technical hurdles to external scrutiny. Here are some common obstacles and practical solutions:
- Data integration and quality: Existing systems often comprise disparate data sources, including legacy systems that do not easily interact. Extracting high-quality data from these varied sources is a challenge due to inconsistent data formats and quality. Implementing robust data management practices, such as data warehousing and data governance frameworks, ensure data quality and consistency. The use of APIs can facilitate seamless data integration.
- Scalability: Predictive business analytics models that perform well in a controlled test environment may not scale effectively across the entire organization. They can suffer from performance issues when deployed on a larger scale due to increased data volumes and transaction rates. Invest in scalable infrastructure, such as cloud-based platforms that can dynamically adjust resources based on demand.
- Regulatory compliance: Financial institutions are heavily regulated, and any analytics tool must comply with existing laws — such as the Fair Credit Reporting Act in the U.S. — which govern data privacy and model transparency.Including explainable AI capabilitieshelps to ensure transparency and compliance in your predictive models. Compliance protocols should be regularly reviewed to align with both internal audits and external regulations.
- Expertise: Predictive analytics requires specialized knowledge in data science, machine learning and analytics. Develop in-house expertise through training and development programs or consider partnerships with analytics firms to bridge the gap.
By addressing these challenges with thoughtful strategies, organizations can effectively integrate predictive analytics into their systems to enhance decision-making and gain a competitive advantage.
From prediction to prescription
While prediction analytics focuses on predicting what may happen, prescription analytics focuses on what you should do next. When combined, you can use the results to optimize decisions throughout your organization. But it all starts with good data and prediction models.
Learn more about Experian’s predictive modeling solutions.
1Experian (2020). Machine Learning Decisions in Milliseconds
*This article includes content created by an AI language model and is intended to provide general information.