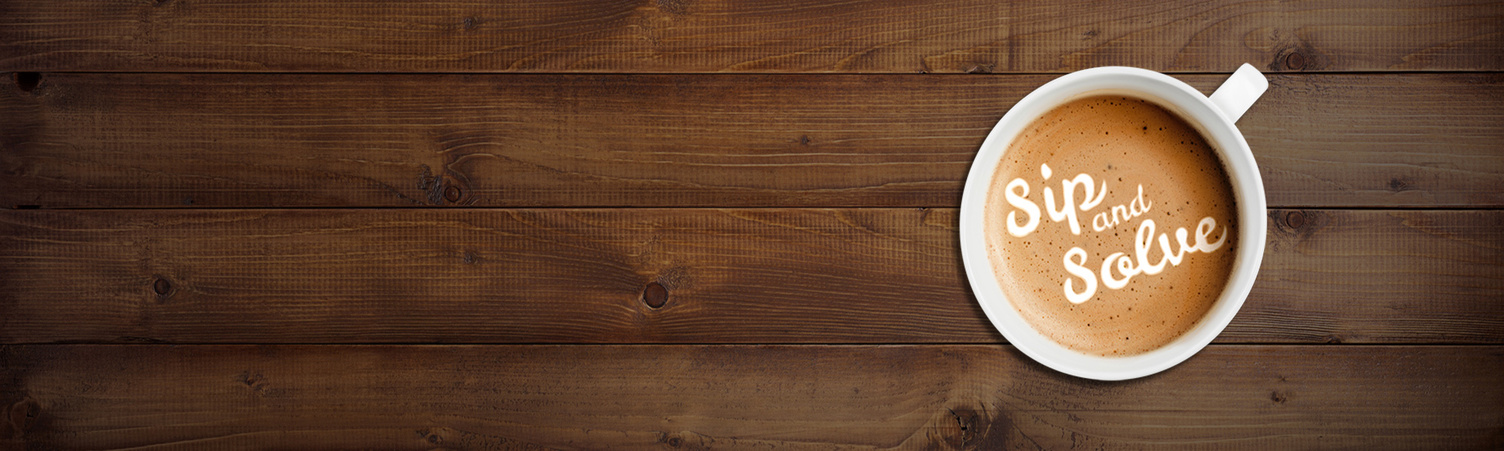
Sip and Solve™
Solving business challenges in a coffee break
As the complexity and sophistication of fraudsters continue to evolve, many banks and financial services organizations might be stuck trying to identify and combat 3rd party fraud with outdated processes. Join us for a Sip and Solve as we share tips on how to identify 3rd party fraud and things you can do today to start modernizing your fraud strategy.
In this jam-packed 15-minute session, learn:
So, my name is Li Mao, I'm a Product Manager here. My product area of focus is commercial fraud. And today, no surprise. We're talking about fraud. I'd really like to consider this, today's session as a continuation as my previous, Sip and Solve session "Combatting Digital Fraud Attacks" with those that did attend last time during the National Fraud Week on combating digital fraud.
If you missed it, and I know we're going to have, I want to rewatch it. I know we're going to have a link available to you. So, I'm going to quickly go over the next two slides as a refresher to. To on what we chatted about in the last video, because really, as I mentioned, it's a continuation, right?
I always like to start with understanding how big the problem is. So, we all know there's a massive amount of data that fraudsters have access to today and data is gold, and they have a ton of it. This is important because it's one of the reasons why we're seeing an increase in the trends. On the very top left-hand corner, what we've seen is 14.4 million identity theft victims. Terms in the U. S. In 2018, 4 billion in losses due to account takeover fraud on the right there. And really, they're using, data that they stole to create identities during the account opening stage, which is why you're seeing about 3.4 billion in a new account fraud loss.
In our last session on combatting digital fraud, the focus was really on implementing business verification at a minimum as a baseline layer to your multi-layer fraud strategy. So, it's a suitable place to start either manually reviewing or through the PI. I've reviewed a number of different data elements. Some of them I have up here. Here on the screen that you should include as part of your business verification, beyond just name and address. So, things I've touched on was business to owner business to consumer linkage, social media to identify knockout rules using geolocation data as well as techno graphics and email.
So today we'll talk more about how these elements are still critical but will be less effective by itself if you're under attack by a third-party fraud.
So, as we saw in the first slide that I mentioned with how big the problem is, right? So, we've seen an increased identity theft and four billion in losses due to account takeover fraud. Today, I really want to narrow that focus and help you think differently and how you tactically could build a better fraud strategy to solve one of the growing fraud types I mentioned previously, and that's third-party fraud.
So, before we can solve that problem, though, I believe it's critical we start to understand what type of fraud you're facing because doing so will provide two things, right? One of the first things it will provide is ability to distinctly classify the type of fraud occurrences because doing so will help you confirm the underlying behavior of the fraud.
Now, the underlying behavior of the fraud is important because it helps you establish a custom-tailored strategy to detect that same type of fraud moving forward. second point really is each type of fraud may require you to create a slightly different strategy. So, understanding that type of fraud will help you also understand what type of Data analytics. and the capabilities you should be utilizing and effectively solving that specific fraud occurrences. First party, third party is differences, and you want to be using different tools to solve this specific type of fraud. It's always about finding the right tool for the right job.
In front of us right now, I have three common third, third party fraud types that I've, heard quite a bit. first one is loan stacking. So, this is multiple credit lines that are being exhausted and payments are stopped simultaneously. So, I really view after loan stacking as the more significant issue. If you're unable to solve the second and third type. or fraud mentioned below, really around the identity.
I mentioned account takeover already. So, this is when fraudsters gain access to an account that doesn't quite belong to them. You may hear or see fraudsters really resurrect dormant businesses by attaching their own identity to the business.
You have a legitimate person or business owner. I would say legitimate in terms of identity. So, they have that identity established. It's a real identity tied to, say, a fake business or a dormant business. Now, that thing boils down to the last fraud type I mentioned here, is that the synthetic ID.
Fraudsters using a real person's name, SSN, but associating with a different address altogether. I’ve seen that address used in a hotel, for example. Now, that's just one scenario of several types of synthetic ID fraud, and we're seeing that within businesses as well. So synthetic Business identities.
We all know that there's a lot of business information out there that's publicly available. So that makes it even easier for someone to perpetrate as a business and the fraud lawsuit we're seeing on the commercial business side. It's much larger, right? You're seeing more volume on the side, but on the commercial side, you're going to be hit for larger losses, and that sounds from a fraudster’s perspective, that's really the right target they're going for. So, some of the key challenges with these types of frauds is that traditional fraud models are just not highly effective because these identities are hybrids of real and fake identification.
Synthetic IDs are not detected by anomaly or common fraud detection tools or controls. Today. They really show up as your best customers. A lot of times because fraudsters, as I mentioned, they're specifically engineering profiles to have strong credit scores and wait patiently waiting to cultivate them over time to gain that higher credit limit or loan amount.
The impact to you is that it's increasing the propensity for higher fraud losses to your portfolio when they are eventually. Bust out, right? There's no silver bullet to identify this type of fraud. We do see a need for deep trended data with advanced analytics required to track that behavior and identify these types of frauds.
And the next couple of slides I'm going to talk about how you could have a chance at really solving that problem.
So, I'm going to cover this slide too quickly because I used in the last session. This is where a strategy I shared in the last video, where you compare the application of information against the things, the data that we do have on file. Bureaus like Experian, right? So, the more you're able to verify corroborate and link things together, the stronger your trust should be that this is a legitimate business and applicant. Now against third party fraud. This approach, while still important, is no longer effective or scalable. So, you need to start thinking about the next phase where you're bringing a more analytical intelligence that I've mentioned to bring in all the disparate pieces that in an automated way.
So, our intention is to by pulling this together, we can start to build a clear identity of a business.
So, here's a great illustration of all the different layers to consider as part of your overall layered approach. So, by taking a layered approach, you could have additional potential fraud trigger events, establishing leading indicators to help you look at the application a little closer before you let them through the door.
It's trying to introduce friction in the potential bad guys you are seeing and letting the ones you know are good guys quickly through the door. So, identity isn't clear cut. It's a combination of multitudes of different attributes. And it's about the relationship between these attributes.
We have a solution today called CrossCore. It's a single platform which allows you to access all of the capabilities that I've listed here on the screen today, as well as Machine Learning models you could access that allows you to quickly adjust and tune your strategy from, in real time with fraud and also a decision layer to bring all the capabilities together.
Now, all these layers make the fraudsters job much more difficult. And they're looking for the path of least resistance. So, the idea is that you start to combine something that they have. so that would be, say, something like a device. So, some common questions we want to be able to answer. Part of the device is, have we seen this device before? Or have we seen them use the device? Are they used in other online applications? What type of device is it? Is the device running an emulator? fraudsters will use emulators to make an Android device look like a phone device. So, this is very sufficient information, right? There's also something called OCR, Optical Character Recognition. That can recognize text and images. So, when you collect a document right now, if you're not utilizing this technology, you're relying on a person reviewing the document to help to review that document themselves to verify that. This by moving into automated way, you would improve that client. experience because as a matter of intervention, I do feel it’s a way to introduce friction.
So, this should help you answer questions like, is this business license legitimate? is the Secretary of State filing legitimate? Or even this passport legitimate?
You combine something what they have with something that they are. So, think of, behavior-based solution. So that's under, so that'd be under say, the first device assessment. Okay. first layer that I have on this slide here, right? So, think of behavior-based solution. This is a person filling out the application. Are they copying and paste a lot of data? Because that's indicative of fraud.
Are they spending more time on a difficult field such as S.S.N for example, that's outside of really industry benchmark. Are they all tabbing on their laptop or their keyboard or not. So, as you start to build in, then, the decision layer on top of the attributes that I mentioned something that they have something that they are. So when you start to pull them together, say, hey, if they're using an emulator and the device configuration, some type of foreign language, and there's a velocity of activity over time, and the person is doing a lot of copy pasting and the email associated has only been around, say, a couple hours now to start to go. Hey, that's suspicious.
So, if you start to see that there are red flags, then you start to then introduce friction into the application process. So, a potential friction you could introduce is a step-up authentication. So, this is when you bring in something that they know. So, things like, what type of mortgage payment does the potential business owner have or what's their last address, for example. So, I'm sure a lot of you have answered these types of questions during the account opening process. So, this is opportunity to incorporate something like the Experian KnowledgeIQ as part of overall fraud strategy.
The idea is then you start to bring in something that they have something that they are something that they know, and then string along. By a decision layer, then it could be more effective. Ultimately, try to identify third party fraud.
So right here I have all of the different elements. So, we're looking for indicators, red flags or knockout rules during your account opening process. Here's a quite simple flow of some of those data sources and tools used to evaluate some any of your incoming business. The fraud data sources that I mentioned the bottom there combined also with the information you're using from credit, right? And then combine that with, say, the document verification, the social media information, device ID, the behavioral things, and the one-time passcodes. Then you're able to bring this together, incorporate into some type of scorecard or strategy workflow, like how I've outlined here.
And then, as I mentioned, to be even more advanced in that, you can even start leveraging supervised and unsupervised machine learning techniques to continually improve that fraud detection over time. The goal also is well, a lot of it's trying to eliminate that false positive because the idea is you want to let all your good consumers through the process easily, but then throw up, but then throw up red flags. To need to have a population that you're viewing and referring, but you want to still have a quick decision for those as well. So, our whole reason for doing this for the small business is to create the most accurate decision quickly, the most accurate as quickly as we can. So, for you as a lender, we want to provide a place for you to manage your entire fraud and identity portfolio in a single platform, reduce the complexity and eliminating the burden of your IT team that must implement all the different data capabilities. and you also have to bring a decisioning layer on top of that, so the complexity of that, trying to reduce the amount of complexities to it, and also have a way for you to adjust the decision strategies on the fly so that you can reduce your outsource or manual review rates and fraud rates.
And then, also experiment more easily with new fraud and identity services as we make them available in our platform.
Again, the idea is to implement a strategy where you could bucket most of your applications into the auto approval, auto decline decision. And that's what on the very left, right?
So, under decision types, you see the very red bucket, the auto decline, and then the green bucket on the very bottom towards auto approval. So, we're trying to fill up those buckets, most of your application population in those buckets. So, I would say a goal to strive for. so, we must manually review about 8 to 10 percent of your overall application population.
So, then that would be in the 8 to 10 population should sit in that middle, right? Your decline refer review, and then the approved refer review. Manual review team should have manually when they review this process, your team should have a 99 percent confidence that once they spend about 30 to 60 minutes investigating, they can readily identify that this is a fraudulent application or not.
Now, the simple thing is, why are we putting so much effort into this process? I think we should have to keep in mind the customer, our end customer, because we want to improve that customer experience. And that's the entire focus of what we do, right? We want to protect the good consumers and small business to help stay healthy and profitable.
We want to reduce fraud coming through the door up front. So, we can eliminate the cost associated after the fact when you lose out on the money that you're lending to them when the goods that you're providing to them as part of a sale. So, with fraudulent activity through our customer lifecycle, we do want to reduce that operational costs and loss that then allows your business to shift your resource focus to really growth and market.
And as a good fraud technology and strategy, as a good fraud technology data analytics partner, we want to easily help you to meet and exceed regulatory requirements with explainable, stable, and predictive outcomes and process. The end goal is for all of us to share in the success of our customers, and we are here to help them and find the capital they need to build a successful business and financial ecosystem that we all work in.
Li Mao is a Product Manager of our Global Data Network, our international offering that supports companies who are operating internationally manage their risk for customer acquisition and across their portfolio. Li is focused on creating a set of tools that will enable professionals to make the best decision in the most efficient way. He is passionate about leveraging new technologies and challenging the norm in order to move the industry forward.
We will be scheduling more Sip and Solve sessions. Sign up below to be notified.
This site is protected by reCAPTCHA and the Google Privacy Policy and Terms of Service apply.